Breaking Down DeepSeek AI: Innovation, Design, and Market Impact
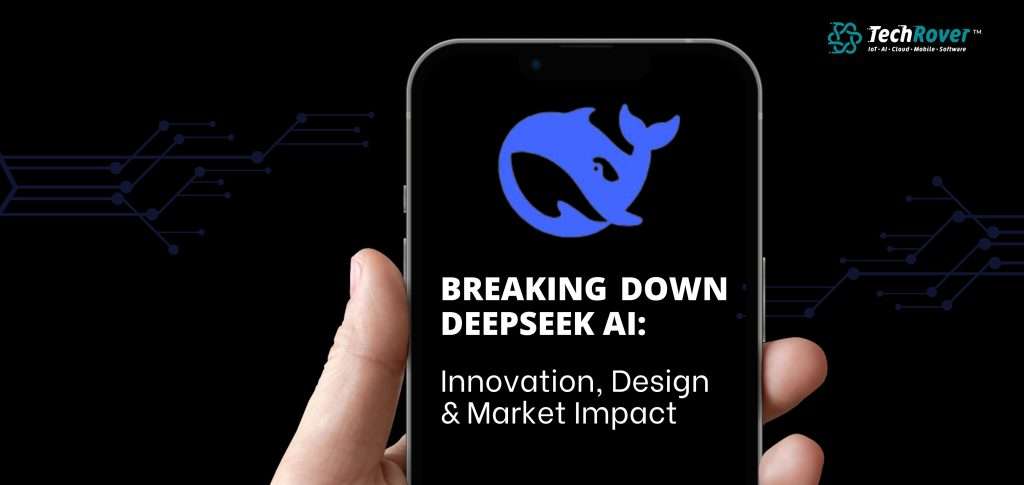
DeepSeek AI is a cutting-edge Chinese artificial intelligence model designed for tasks such as text generation, language translation, and human-like conversation. Developed to rival leading AI technologies from OpenAI, Google, and Meta, it represents China’s push toward innovation and self-reliance in AI.
Trained on large-scale datasets, DeepSeek AI excels in handling complex queries, making it highly effective for content creation, question-answering, summarization, and chatbot applications. As China continues to advance its AI research, DeepSeek AI marks a significant step in reducing dependence on Western AI systems.
Model Architecture
The basic architecture of DeepSeek-V3 is still within the Transformer (Vaswani et al., 2017) framework. For efficient inference and economical training, DeepSeek-V3 also adopts MLA and DeepSeekMoE, which have been thoroughly validated by DeepSeek-V2.
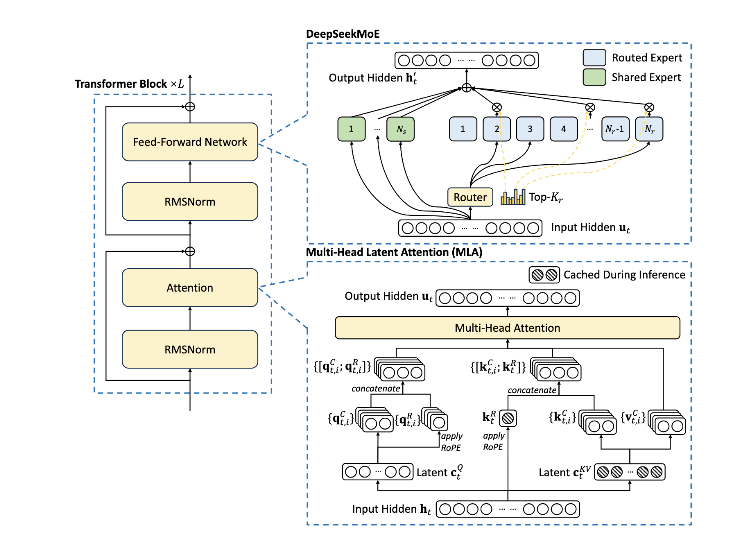
Key Architectural Innovations
1. Mixture of Experts (MoE) Framework
- Utilizes an MoE-based architecture where specialized “expert” subnetworks handle different computations.
- Dynamically selects a subset of experts per token instead of using all parameters, reducing computational costs.
- Enables efficient scaling while maintaining resource-efficient inference.
2. Multi-Head Latent Attention (MLA)
- Optimizes attention mechanisms, first introduced in DeepSeek V2.
- Enhances inference speed and memory efficiency.
3. DeepSeekMoE for Training Optimization
- Improves training efficiency by distributing workloads across experts.
- Reduces imbalances that could impact model performance.
4. Load Balancing Strategy
- Addresses uneven expert utilization, a common challenge in MoE models.
- Implements an auxiliary-loss-free balancing strategy to optimize expert activation without performance trade-offs.
5. Multi-Token Prediction (MTP) Training
- Predicts multiple tokens in parallel instead of one at a time.
- Increases training efficiency and speeds up inference.
6. Memory Optimization for Large-Scale Training
- Eliminates the need for tensor parallelism, reducing memory and computing resource requirements.
- Improves training efficiency on GPUs, enabling cost-effective large-scale deployments.
Competitive Advantage
- Achieves strong performance with lower training and inference costs.
- Positions DeepSeek V3 as a competitive open-source alternative to models like GPT-4o and Claude-3.5.
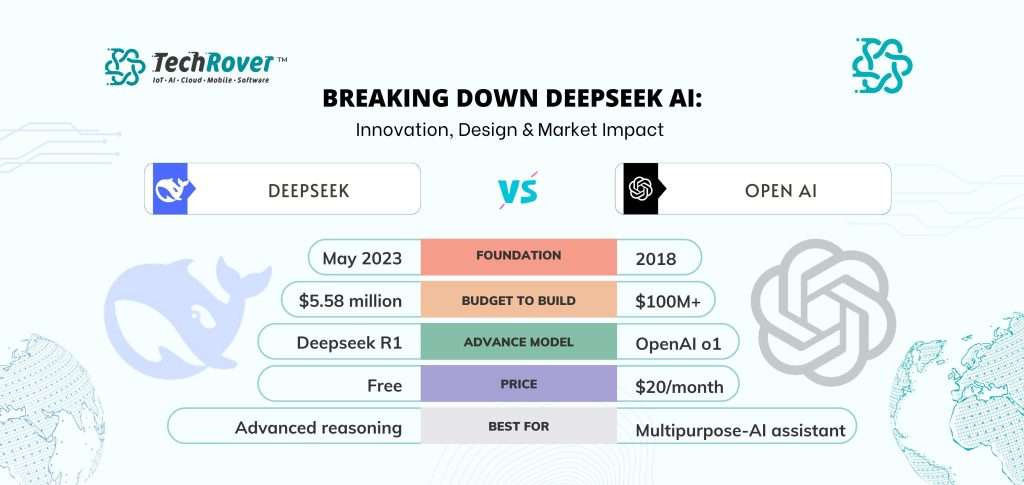
Performance Highlights
1. State-of-the-Art Performance
- Outperforms open-source models in knowledge, reasoning, coding, and math benchmarks.
- Competes closely with GPT-4o and Claude-3.5 in multiple evaluation metrics.
2. Benchmark Scores
- MMLU: 88.5
- MMLU-Pro: 75.9
- GPQA: 59.1
- Surpasses other open models in general knowledge and reasoning tasks.
3. Math Excellence
- Outperforms OpenAI’s o1-preview on MATH-500.
4. Coding Dominance
- Ranks highest on LiveCodeBench, demonstrating superior coding capabilities.
Performance Comparision:
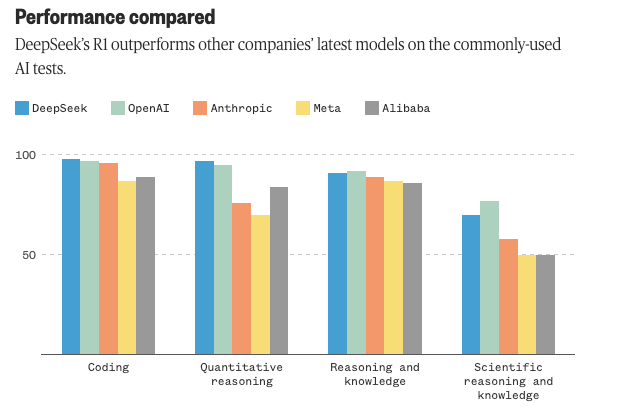
Comparative Analysis: DeepSeek AI vs. ChatGPT, Claude, and Gemini in Generative Tasks
Evaluation Approach
To assess the real-world performance of DeepSeek AI against industry leaders ChatGPT, Claude, and Gemini, we conducted a standardized test focusing on generative capabilities.
- Each model was prompted with the same request: Generate an image of a candle with a flame.”
- To ensure a fair evaluation, minimal prompt engineering was applied.
- The test was conducted across multiple runs to measure consistency, detail retention, and contextual accuracy in image generation.
Key Findings
- DeepSeek AI exhibited superior spatial coherence, diffusion stability, and structural accuracy, generating images that closely adhered to real-world physics.
- Claude & ChatGPT demonstrated strong semantic understanding, though they showed minor inconsistencies in object boundaries and light diffusion.
- Gemini struggled with object persistence and illumination fidelity, occasionally misinterpreting key visual cues.
- Our analysis indicates that DeepSeek AI’s diffusion-based model architecture is optimized for higher object fidelity and precise prompt adherence, positioning it as a strong contender in precision-driven generative AI tasks.